Leveraging AI for Information Management: Striking a Balance Between Benefits and Risks
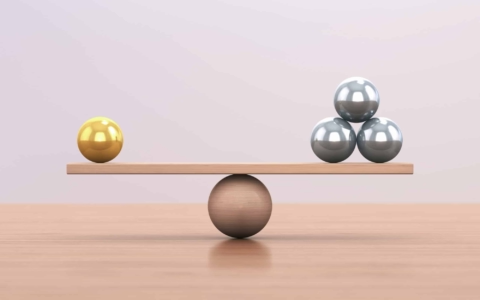
Information management and artificial intelligence (AI) can work powerfully together — when done thoughtfully.
In our recent webinar, Information Management and AI: The Good, the Bad, and the Mildly Unattractive, Alyssa Blackburn, AvePoint Director of Records & Information Strategy, and Bruce Berends, AvePoint Product Strategy Lead, explored how organizations can tap into AI’s advantages while avoiding its potential downsides.
Read on for a complete recap of their insightful discussion or watch the conversation on-demand.
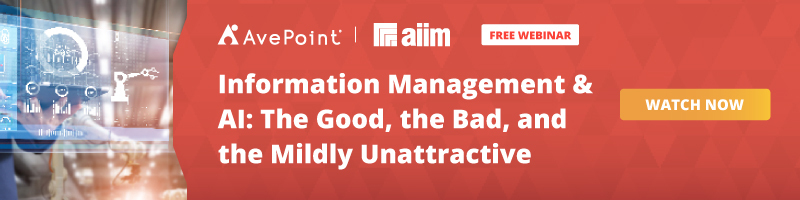
Why Information Management Matters — A Quick Recap
Before diving into AI, Alyssa provided a quick recap of why solid information management is so important for organizations today. Effective information stewardship helps:
- Ensure information integrity
- Drive efficiency
- Provide cost savings
With exponential data growth overwhelming traditional practices, approaches to information management must be modernized to deliver effective outcomes reliably. Strong data quality and governance will boost an organization’s ability to maximize technologies like AI.
Mapping Out Key AI Categories: Generative vs. Process-Focused
AI solutions typically fall into one of two broad classes:
- Generative AI: Technologies like ChatGPT and Microsoft 365 Copilot that can create brand-new content and materials (text, images, video, presentations, etc.)
- Process AI: Technologies that optimize and enhance existing business operations through automation, prediction, data analysis, etc.
Rather than positioning these two categories as rivals, Alyssa and Bruce emphasized their potential symbiosis. Organizations should match specific AI solutions to targeted use cases, not assume one approach can meet every need.
Harnessing Process AI: 5 Key Advantages
When boosted with tools like machine learning, robotic process automation (RPA), text analysis, or document fingerprinting, your information management program can be elevated in five game-changing ways:
- More Efficient Data Handling: AI can automate data extraction from documents; classify based on keywords, metadata tags, and the like; and organize into appropriate storage structures – all reducing manual effort and cutting process costs.
- Enhanced Content Search and Retrieval: Context-aware AI search engines simplify finding relevant information across massive document collections and disparate systems. This saves end users tremendous time. Research finds nearly 50% of employees can’t find the work documents they need. So, enhanced search and retrieval abilities unlock major productivity gains.
- Higher Overall Data Quality and Accuracy: AI pattern recognition helps detect errors and inconsistencies, identify duplicate records, and flag outdated information. Feeding cleaned datasets back into business systems improves operational reliability.
- Substantial Cost Reductions: By automating repetitive tasks, process AI cuts demands on human employees massively. This translates into lower operational costs and enables staff redeployment to higher-value activities.
- Data-Driven Decisions and Insights: Sophisticated analytics, powered by machine learning algorithms, expose invisible trends and relationships in data. This amplifies human analysis to drive faster, smarter choices.
Hear from Alyssa about her take on how to achieve business value, the use case for AI, and the measurable productivity, efficiency, and cost-saving gains you can achieve by incorporating it into your information management strategy.
Implementing Process AI Effectively
While the advantages of process AI seem compelling, Bruce and Alyssa underscored several key considerations for getting real rather than theoretical benefits:
- Don’t Confuse Process Automation with Intelligence: Straightforward automation differs fundamentally from context-aware, self-learning AI. Process workflows lacking adaptive decision-making produce suboptimal (or even harmful) results despite high efficiency. Australia’s infamous “RoboDebt” scandal exemplifies the perils of prioritizing process over accuracy in public sector administration. Faulty income-averaging algorithms produced hundreds of thousands of incorrect welfare payment notices — a massive failure tracing back to blind automation without data accuracy or oversight.
- Continually Re-Evaluate Potential Outcomes: Rather than fixating on possible tools, anchor process innovation in achieving measurable operational outcomes. What challenges does the organization need to solve? Outcome-oriented thinking also helps match the right solutions to the right use cases while steering clear of “shiny object” syndrome claims that AI can magically fix any problem.
- “Garbage In, Garbage Out” Still Applies: No technology bypasses underlying data quality challenges. Attempting to extract valuable insights from “dirty” datasets polluted with errors, redundancies, and inconsistencies generates more bad outputs. Resist overclaiming AI’s benefits until rigorous data governance is in place.
- AI Is Never “Set and Forget”: While touting AI’s low maintenance might sell products, the truth is quite different. After initial implementation, models need continuous monitoring to align with business objectives, regular accuracy checks, and refinement and retention based on changes in the environment. For long-term reliability and impact, organizations must budget for ongoing iteration informed by usage in production systems.
- AI Complements (Not Replaces) Data Governance: Rather than weakening existing quality standards, process automation makes upholding them at higher volumes and complexities more feasible. In parallel, insight generation creates opportunities to identify data disconnects and rationalize policies moving forward. AI works best by augmenting people, not replacing them.
Hear from AvePoint Co-Founder and CEO Dr. Tianyi Jiang on How to Unlock the Power of AI with a Robust Data Strategy
Tying Processes to Outcomes: A Case Study
Alyssa and Bruce provided a real-world example from an AvePoint public sector client grappling with imposing content archives after a legacy system migration:
Without understanding what documents resided in these massive sets – let alone where specific files existed – the backlog severely slowed business operations dependent on records retrieval. Manually indexing thousands of documents proved impossible with existing staffing.
With AvePoint, however, the organization trained models to recognize 14 categories of high-priority documents critical for daily operations (and legally mandated availability). This automated classification within the legacy archive massively decreased costs and risks related to non-compliance while increasing productivity and decision reliability.
Additional examples covered demonstrating process automation opportunities:
- Streamlined Accounting: Classifying and extracting key data from invoice documents to accelerate downstream invoice processing.
- Enhanced Logistics Processing: Distinguishing invoices from superficially similar shipping documents to minimize erroneous handling.
For each, Alyssa and Bruce emphasize considering desired outcomes before implementing technology solutions. Doing so helps focus efforts, justify resource allocation, and sequence holistically versus getting distracted by individual capabilities.
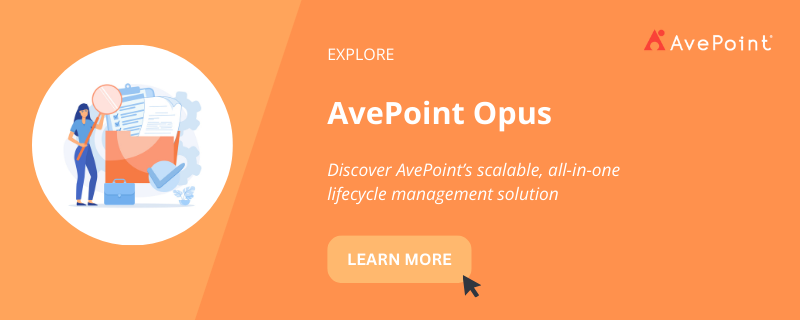
Evaluating Generative AI: The Good, Bad, and Mildly Unattractive
While most coverage focuses on impressive but narrow applications like conversational chatbots, generative AI’s long-term potential spans much further across enterprises — alongside equal measures of exhilaration and concern.
The Good
Let’s start with the upside of AI:
- Lightning-Fast Content Creation: Generative AI models can create readable long-form content, emails, code, and more in seconds that would take humans hours or days. This unlocks new efficiencies for writers, legal teams, programmers, and anyone producing materials manually.
- Sparking Creativity & Enriching Ideation: AI content generation amplifies free association and creates novel combinations to enhance individual and group brainstorming. Unexpected phrasing prompts and unconventional perspectives stretch thinking.
- Customization and Personalization: With infinitely variable inputs and production scalability, AI-generated text, imagery, and other assets can hyper-target specific user niches and usage contexts vs. one-size-fits-all.
The Bad
Here are potential risks that must be accounted for:
- Privacy, Security, and IP Vulnerabilities: Language models ingest gigantic volumes of text and data to discern patterns, including blocks of copyrighted and private material, scraped websites, sensitive communications, and more, with limited consent. Generative outputs risk amplifying this exposure.
- Propagating Misinformation and Biases: Despite engineering priority shifts after high-profile stumbles, subtle model biases persist, such as portraying demographic groups offensively or reinforcing harmful stereotypes.
- Perpetuating Outdated/Incorrect Information: Dynamic language model capabilities don’t guarantee fresh, accurate outputs. Generative AI risks recycling older articles and materials potentially containing superseded facts and figures or policy positions unless underlying training data inventories stay current.
The Mildly Unattractive
As we continue to understand the potential and risks of AI, we must consider the following:
- Difficulty Determining Quality and Accuracy: With models producing such voluminous, human-like outputs, exhaustively fact-checking and contextualizing every piece burdens even large teams. Risks emerge putting AI-generated raw content directly into production systems and processes without verification.
- AI Still Requires Ongoing Human Supervision: While autonomous-sounding capabilities evoke images of set-and-forget systems running themselves, delivering responsible generative AI necessitates continuous, labor-intensive model and output audits, tweaks, and safeguards. Organizations can’t take roles or responsibilities lightly even after launch.
Deploying Generative AI Responsibly
While generative AI adoption seemingly accelerates daily in the headlines, prudent organizational deployment takes greater care and planning. Core considerations include:
- Activating Monitoring, Logging, and Output Tagging: Watermark programmatically generated content for traceability. Log usage statistics, runtime inputs, and key metadata for trend analysis.
- Curating High-Quality Inputs: Continuously groom datasets to maximize quality and minimize inappropriate recommendations. Prioritize recent, accurate materials reflecting updated policies and positioning.
- Implementing Human Review Processes: Spot-check outputs to confirm alignment with expectations before dissemination or usage in critical workflows. Create exceptions reporting for unusually long runtimes or off-brand responses.
- Preserving Strong Information Governance: Maintain information lifecycle to prune content that is no longer required for business purposes so that AI only runs across the most relevant and up-to-date content.
Maintaining high underlying information quality remains imperative, even as AI propels its exponential growth. Thinking holistically about people, process, and technology transformation is vital.
Key Takeaways and a Practical Path Forward
Alyssa and Bruce concluded the session with practical guidance applicable across applications:
- Remember Objectives: Ground explorations and experiments in discrete operational outcomes vs. loose AI fascination. What pressing pain point or golden opportunity does AI address across people, processes, and technology company-wide? Progress flows from anchoring efforts to measurable goals reflecting stakeholder needs.
- Start Small, Think Big: Don’t fixate on solving every problem the first day you deploy. Target controlled, low-risk pilots delivering quick, tangible wins. For example, help frequent meeting organizers auto-generate consistently formatted agendas and notes instead of amorphous “AI transformation.” Small starts that seed future ambition keep the momentum going.
- Stick to Basics (For Now): Similarly, focus initial automation on repetitive tasks with a bounded scope, like customer thank you notes or weekly reporting templates. Once processes normalize, progressively expand complexity. Avoid overengineering unproven infrastructure for advanced applications unlikely to materialize soon.
- Keep Humans in the Loop: AI works best by complementing people, not replacing them outright. Build in checkpoints for cross-checking and verification by subject matter experts positioned to discern nuance. Also, create direct feedback channels informing model refinement over time for greater precision.
The Bottom Line
AI holds immense possibilities for information-driven organizations, but realizing benefits demands diligence. Avoiding hype cycles and thoughtfully adopting new capabilities balanced with a pragmatic perspective lets teams selectively support information flows and decisions positively – without getting burned.
Small starts grounded in user needs help unlock advantage while building engagement and enthusiasm for sustainable change. So rather than passively monitoring hype cycles, proactively scope possibilities against operational priorities. Thoughtful ideation around people, process, and technology helps cut through noise and deliver on AI’s promise.
Catch up on the full conversation with Alyssa and Bruce by watching the webinar on-demand today.
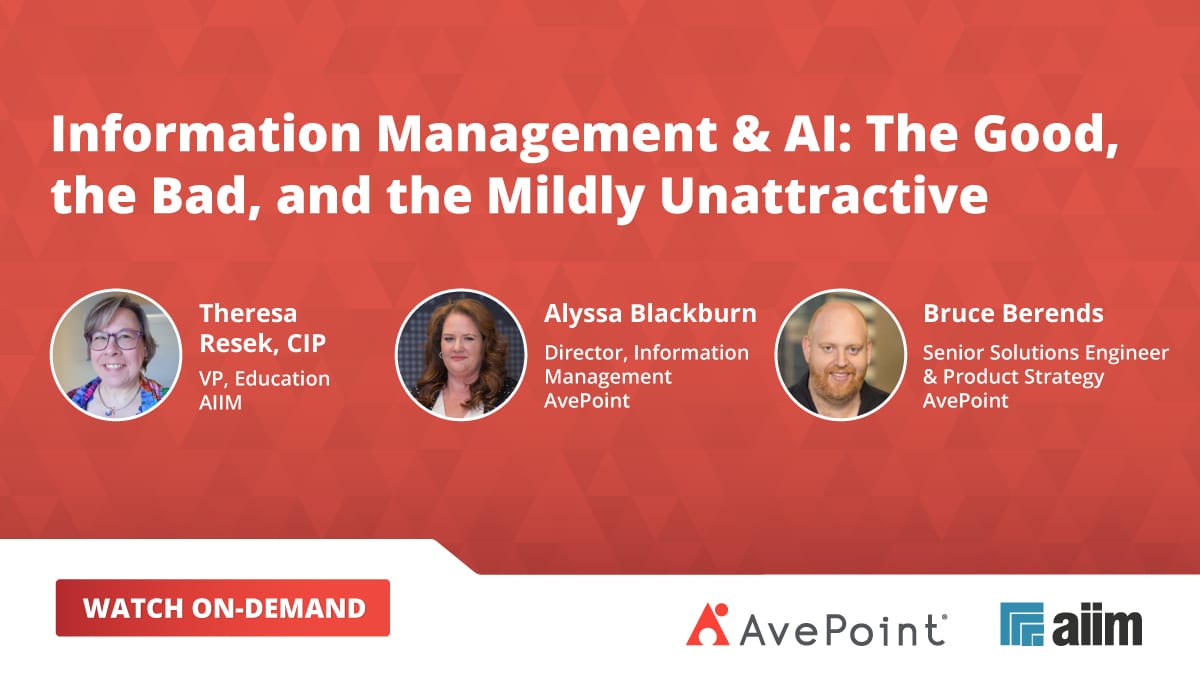
As Senior Director of Content & Communications at AvePoint, Chris is responsible for all external and internal corporate marketing communications. Chris brings more than 15 years of experience to his role at AvePoint, previously holding roles at EisnerAmper, BASF, MetLife and CRM Magazine. Chris received two American Society of Business Publication Editors (ASBPE) awards for feature articles on salesforce.com and generational trends.