In the pursuit of AI's transformative potential, many companies are facing unexpected hurdles. According to a Gartner report titled The Top 10 “Gotchas” of Microsoft 365 Copilot, only 24% of respondents piloting Microsoft 365 Copilot are planning a large-scale rollout in 2024/25—indicating a significant gap between ambition and execution.
Surprisingly, it may not be a lack of technology or vision that's holding them back—but their own data. A recent study by AvePoint, the AI & Information Management Report, found that businesses often find themselves stymied by the quality, accessibility, and governance of their data assets.
Do these challenges sound familiar in your organization? You're not alone - 95% of the organizations in the AvePoint study experienced challenges during AI implementation. The silver lining? There are actionable steps you can take to overcome these barriers and facilitate successful AI integration. Let’s dive into the study's findings and explore strategies for advancing your company's AI objectives.
The Crux of the Matter: Data Readiness
According to the study, while a significant 80% of survey respondents believed their data was AI-ready, more than half encountered internal data quality issues during AI model and application deployment. This glaring disparity underscores a significant gap between perceived readiness and actual readiness in AI implementation.
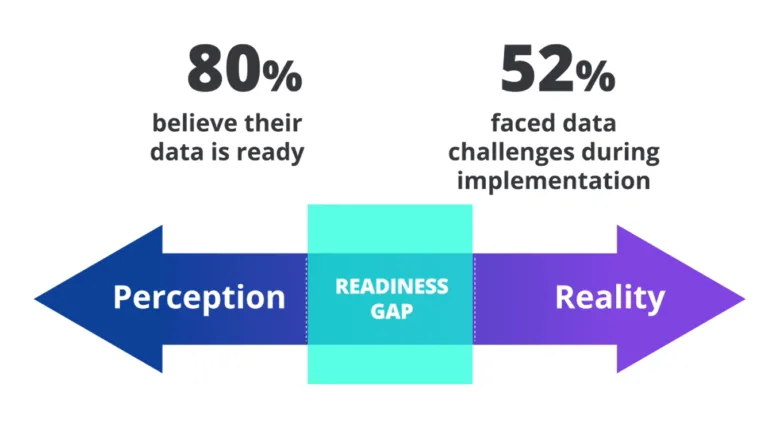
What's behind this discrepancy? It often boils down to a fundamental misunderstanding of what constitutes AI-ready data. AI-ready data must adhere to specific criteria—it should be accessible, relevant, and contextualized. However, the study found that respondents' data often fell short of these standards.
Many companies' storage architectures may not be optimized for AI usage. While 87% of survey respondents stored data in the cloud, many also utilized self-hosted storage (51%) and physical documents (46%). This fragmented data landscape poses a challenge for both humans and AI algorithms, as relevant information may be scattered across disparate repositories, hindering accessibility and effective model building.
Additionally, survey findings revealed 50% of organizational data was over 5 years old, potentially containing redundant, obsolete, or trivial (ROT) data. This not only burdens storage systems but also jeopardizes the integrity of AI-driven insights, which may rely on outdated information.
Finally, respondents cited numerous challenges, such as overwhelming search results, indicating inadequate data categorization and contextualization. This lack of organization makes it difficult to extract relevant information from granular search results, hampering AI utilization.
Organizations must confront the harsh reality that their data may not be as AI-ready as they believe. Many data repositories are plagued by inconsistencies, inaccuracies, and compliance issues, undermining the effectiveness of AI algorithms and impeding growth opportunities. Organizations must act swiftly to address these data quality issues and implement robust strategies for enhancing data readiness to fully unlock the potential of AI in driving innovation and growth.
Refining Your Information Management Strategy is Key
Realizing that your data may need some work to become AI-ready prompts a crucial question: what steps can you take to address this challenge? Think of it this way: much like refining your culinary techniques enhances the dish, honing your information management strategy is key to preparing your data for effective AI utilization.
Quality data is built by effective information management, yet many organizations may not have as effective strategies as they think. Despite claims of robust approaches, nearly half of the study’s survey responses lacked basic information management measures like archiving policies, data retention rules, and lifecycle management solutions.
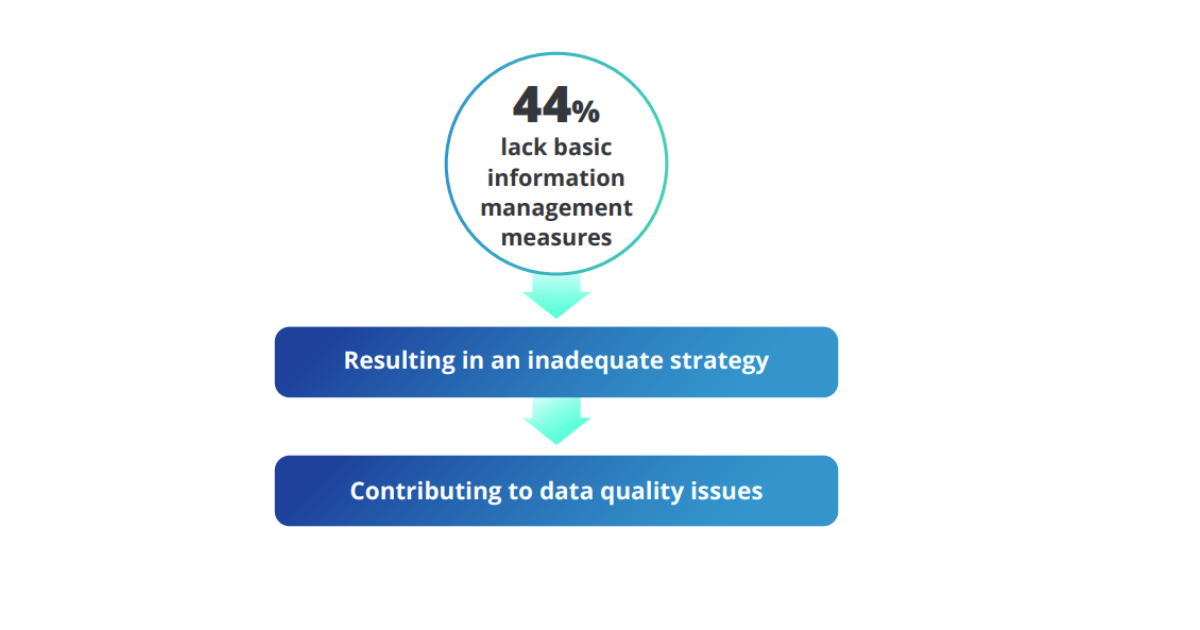
Neglecting these fundamental components of information management not only compromises data quality and integrity but severely undermines successful AI adoption. This was seen clearly in the report: those with less mature information management strategies were not only less confident in their data readiness for AI but also more doubtful about AI’s potential impact on their business.
The bright side: organizations with mature data strategies are 1.5 times more likely to achieve advantages such as improved efficiency, enhanced decision-making, and optimized resource allocation from their AI investments. Learn how to build your effective information management strategy.
Enhancing your information management strategy isn't just about ticking boxes on a checklist. It requires a holistic approach that encompasses people, processes, and technology. It must be an organizational-wide paradigm shift that elevates data stewardship to the same level of importance as any revenue-generating, customer-facing, or compliance-related activity. Automation can play a pivotal role in this regard, particularly as corporate data repositories continue to grow, by reducing manual effort and enhancing efficiency while minimizing the risk of human error.
By addressing these facets of information management, organizations can lay a solid foundation for AI integration and unlock the full potential of their data assets. It's not just about preparing your data for AI—it's about building a resilient and agile information ecosystem that drives innovation and competitive advantage in the digital age.
Don’t Let the Promise of AI Distract You
The study respondents admitted they had concerns about AI before implementing it; 71% were concerned about data privacy and security and 61% were apprehensive about the quality and organization of internal data. Yet many – in fact, the majority of study respondents – still moved forward with their AI initiatives.
This contradiction points to a blind spot; organizations are so eager to reap the benefits of AI, they are willing to put aside their concerns and even knowledge of real risks. Others also fear lagging behind competitors, with 3 out of 4 C-suite executives fearing potential business obsolescence if they don't adopt AI within the next five years.
However, don’t take this to mean you should avoid AI altogether. Instead, it underscores the importance of acknowledging and addressing the uncertainties and obstacles associated with AI before diving head-first into adoption. As we’ve seen here, one of the top challenges you must address is your company data.
Confronting this data dilemma demands a fundamental mindset shift. Rather than an afterthought, information management must become a strategic imperative for effective AI adoption. This necessitates a holistic evaluation of existing practices, plugging gaps, and implementing comprehensive data quality, security, and lifecycle management strategies.
Fostering a culture of data stewardship while leveraging automation and AI-powered data management solutions can transform organizations' data landscapes, ensuring accuracy, relevance and compliance—the cornerstones of AI readiness.
The Time to Act is Now
The AI & Information Management should serve as a wake-up call for leaders: realizing AI’s full potential hinges on robust data readiness. The ramifications of overlooking it extend far beyond operational inefficiencies. As AI becomes increasingly intertwined with critical processes, the accuracy, and integrity of data take on heightened significance. Unreliable or outdated information can bead to flawed decision-making, compromising strategic initiatives.
For leaders intent on harnessing AI’s power, the imperative is clear: prioritize information management as a strategic drive and open doors to a future where innovation, efficiency, and productivity intersect. Remember, in today’s AI era, data reigns supreme – and is a precious resource unlocking AI’s possibility.
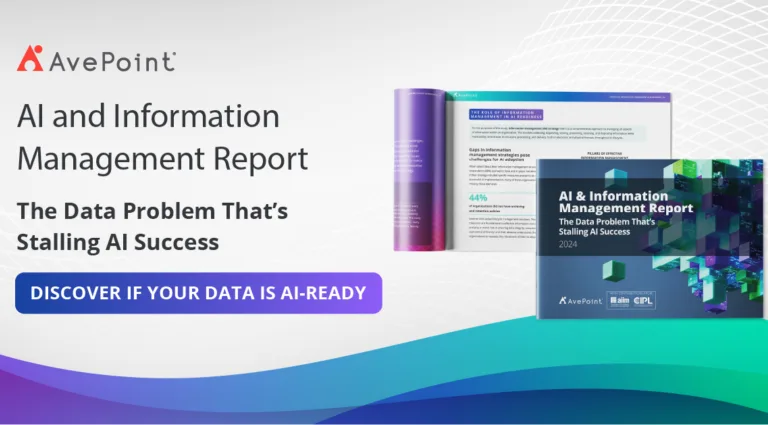