A PwC report estimates that AI could add up to $15.7 trillion to the global economy by 2030, potentially surpassing the combined current outputs of China and India. However, to capitalize on this potential, businesses need a comprehensive data strategy that supports AI innovation, ensuring data is not only available but used effectively to solve problems and create value.
This article explores how businesses can strategically leverage data to enhance AI capabilities while addressing the challenges of data security, governance, and privacy.
Building a Data-Driven AI Strategy
Data plays a pivotal role not just in AI development but also in its practical application. A strong data strategy starts with a clear understanding of key business processes and decision points that AI can augment or automate. Companies should map out these workflows to identify where AI can add the most value, pinpointing the data required for training and refining their AI models.
In the retail sector, 75% of executives view GenAI as crucial for their businesses’ revenue growth, and 93% of retail CxOs plan to scale up their investment in AI over the next three to five years according to research from Accenture. AI adoption is primarily driven today by use cases in marketing and sales, product and service development, and IT. Retailers are using AI for personalized marketing, inventory management, and customer service enhancements. For instance, retail giant Amazon uses AI to enhance customer experience with personalized recommendations. Amazon collects data on purchase history, browsing patterns, and even feedback to fuel its AI systems. This approach enables the company to continuously refine its recommendation engine, driving higher engagement and sales.
A useful framework, as suggested by PwC, classifies AI applications into four categories based on the complexity of decisions and their business impact:
- Efficiency: AI automates routine tasks like data entry or scheduling, reducing errors and improving productivity.
- Effectiveness: AI enhances complex processes such as fraud detection by improving accuracy.
- Expertise: AI supports high-impact decisions, such as customer service, by offering personalized insights.
- Innovation: AI drives innovation, like product development, by generating new opportunities and insights.
By understanding these areas, businesses can tailor their data strategy to unlock maximum AI potential.
Overcoming Data Challenges for AI Success
While data is abundant, using it effectively presents unique challenges. Companies often face hurdles like poor data governance, a lack of analytics expertise, and increasing privacy concerns.
- Data Governance & Quality
Data fragmentation and inaccuracies can result in flawed AI outcomes. For instance, AI in the healthcare industry must rely on accurate patient data for diagnostics. A recent study highlighted an issue with a healthcare company’s AI model which was designed to predict sepsis. The model had a high rate of false positives and missed many actual sepsis cases, which was largely due to fragmented and incomplete data.
- Data Skills & Culture
Many organizations struggle with a lack of data literacy. To truly become data-driven, companies need to foster a culture of data fluency across their workforce. This challenge is similar to what companies like GE Aerospace faced when integrating AI into their operations. By investing in data skills training, they transformed their staff into AI-empowered workers who optimized operations significantly enhanced the accuracy and efficiency of preventative maintenance efforts and advanced sustainability to optimize fuel efficiency and reduce carbon emissions during flight operations.
- Data Security & Privacy
As data becomes more valuable, so does the need for stringent security measures. Businesses face heightened risks of data breaches, which could derail AI efforts. In 2023, data breaches increased by 20% compared to the previous year – largely caused by cloud misconfigurations, new types of ransomware attacks, and increased exploitation of vendor systems – all of which can significantly disrupt AI initiatives by compromising the integrity and availability of critical data.
Realizing the Benefits of Data for AI
When data is used effectively, AI can unlock significant business value:
1. Boosting Productivity
AI streamlines business operations by automating manual tasks. For example, Siemens uses AI-powered systems to optimize factory operations, reducing machine downtime and improving productivity by up to 20%.
2. Improving Customer Satisfaction
AI helps businesses provide personalized services, leading to better customer engagement. Netflix is a prime example, using data to personalize content recommendations and produce original shows based on viewer preferences, resulting in improved customer retention.
3. Driving Innovation
Data-driven AI fosters innovation. Fashion service Stitch Fix uses AI to offer personalized styling, analyzing customer preferences to recommend outfits. This innovative use of AI has earned the company a loyal customer base and a competitive edge in the market.
Conclusion: Data as the Key to AI Success
Data is the foundation upon which successful AI systems are built. By developing a strong data governance framework, cultivating a culture of data literacy, and safeguarding data privacy and security, businesses can maximize the value of AI. Those that effectively manage and use data will not only enhance their AI capabilities but also create lasting value and competitive advantage.
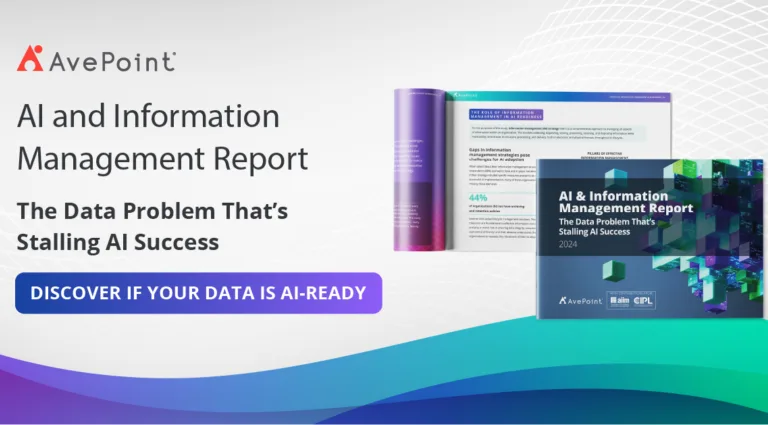