The MOVEit Transfer data breach affected numerous organizations and exposed sensitive data, causing widespread concern and financial loss. This incident underscores the dual-edged sword of AI: its potential to revolutionize industries and the critical need for robust security measures. Imagine a world where your personal assistant knows your every preference, your car drives you safely to your destination, and your medical diagnosis is delivered with pinpoint accuracy — all thanks to artificial intelligence (AI).
However, with great power comes great responsibility. The same AI systems that enhance our lives also pose significant data security challenges. A single data breach can expose sensitive information, damage reputations, and cause financial losses. That’s why organizations must do more to prepare and secure their data in the age of AI.
The Importance of Data Security in AI
Without data, AI cannot function. These systems depend on vast amounts of data to learn, reason, and act. However, this data is susceptible to theft, misuse, and manipulation. Data breaches can reveal sensitive information, tarnish reputations, and result in financial losses. Misusing data can infringe on privacy and violate ethical and legal standards. Manipulating data can undermine the integrity and reliability of AI outputs, leading to biased, inaccurate, or harmful decisions.
Last year, organizations experienced a 72% increase in the number of data compromises over the previous record of 2022. This year has been no different, with 1 billion records stolen (and counting) as of August including AT&T, Ivanti, Change Healthcare, and more.
As we continue to witness an alarming rise in data breaches, with over 1 billion records stolen this year alone, the need for robust data protection measures becomes ever more critical. Ensuring the integrity, privacy, and security of data is not just a technical necessity but a moral imperative. By safeguarding our data, we protect the foundation upon which AI systems are built, ensuring they remain reliable, ethical, and beneficial to society.
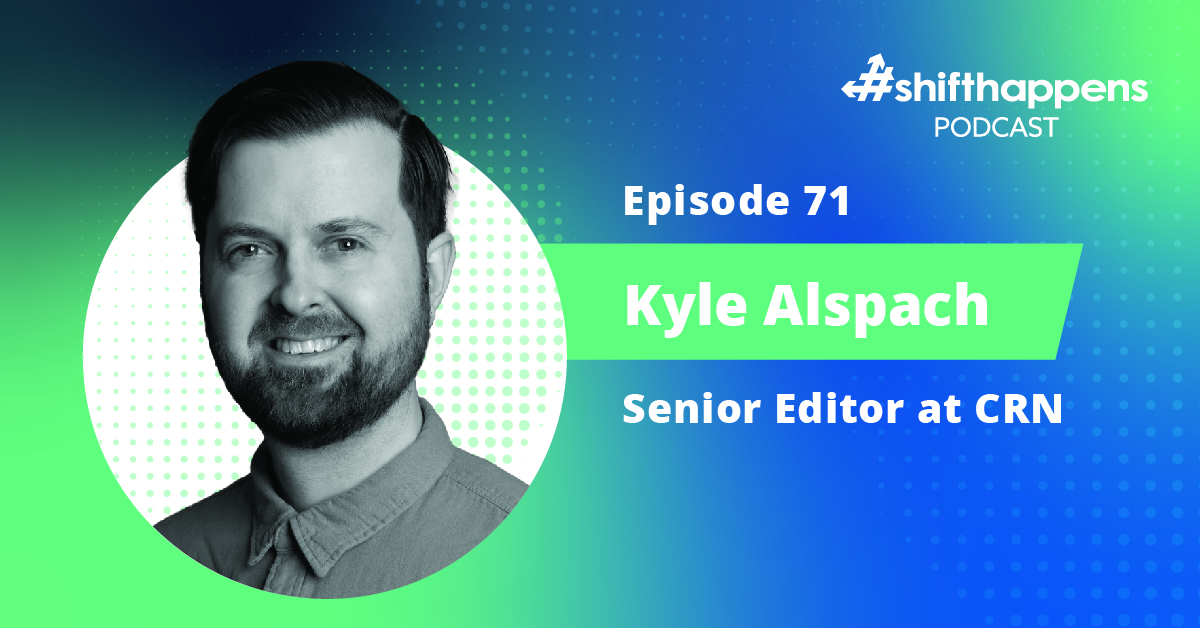
Security Measures for AI
AI security is not a one-size-fits-all solution. Different AI applications have varying security requirements and implications. Therefore, adopting a risk-based approach to find and prioritize potential threats and vulnerabilities is crucial.
Consider the type and sensitivity of data used by the AI system. Personal, financial, or health data may require higher levels of protection than public or anonymized data. For instance, an AI system handling medical records must ensure stringent data protection measures to maintain patient confidentiality and comply with regulations like HIPAA.
The purpose and function of the AI system also plays a significant role. AI systems performing critical tasks, such as medical diagnosis or autonomous driving, require higher levels of accuracy and reliability. A misdiagnosis or a malfunction in an autonomous vehicle can have life-threatening consequences, making it imperative to implement rigorous testing and validation protocols.
The context and environment in which the AI system works are equally important. AI systems in highly regulated or competitive domains, such as banking or defense, face higher levels of scrutiny and compliance. These systems must adhere to industry standards and regulatory requirements to ensure their security and reliability.
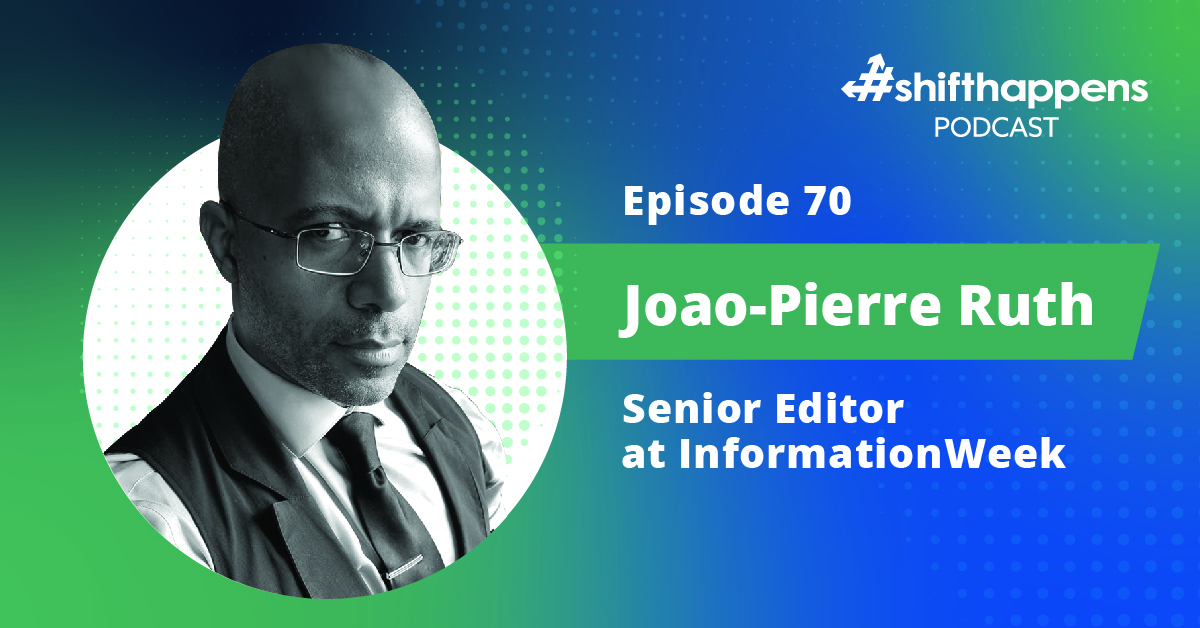
Ethical Implications of AI
Ensuring AI systems adhere to principles of fairness, accountability, and transparency is essential. Is the AI system fair and unbiased? Does it avoid discriminating or harming certain groups or individuals? These questions are crucial in evaluating the ethical implications of AI.
Accountability and responsibility are also key considerations. Are there mechanisms to monitor, audit, and explain the AI system's actions and outcomes? For example, an AI system used in hiring processes must be transparent about its decision-making criteria to avoid biases and ensure fair treatment of all candidates.
This was the issue Mona Sloane, a senior research scientist at the NYC Center for Responsible AI, delved into when helping support a New York City law which went into effect last year restricting the use of AI tools in the hiring process. In her recent research, Sloane found AI systems meant to measure personality aren’t reliable. While not all companies use personality assessment tools as part of their hiring processes, she shared concern about when these tools are integrated without the knowledge of recruiters or applicants.
Transparency and explainability are vital for building trust in AI systems. Does the AI system show its data sources, methods, and assumptions? Providing clear and understandable reasons for its decisions and recommendations can help users trust and accept AI-driven outcomes.
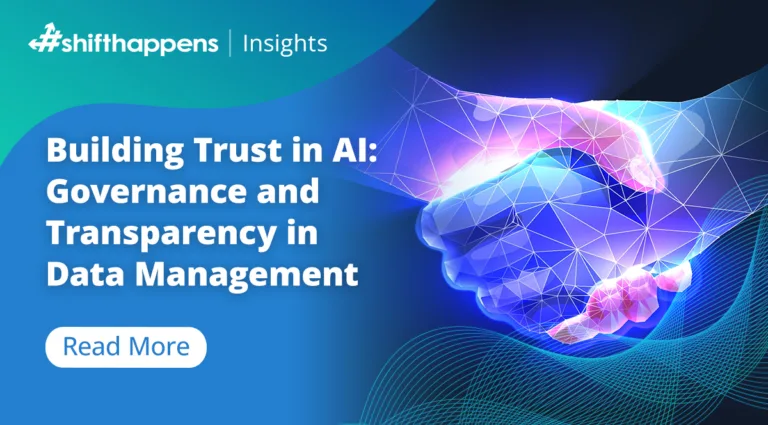
Best Practices for AI Security
Enhancing the security of AI systems is a multifaceted challenge that requires a comprehensive approach. Based on insights from PwC and other industry experts, several best practices can be recommended to bolster AI security. These practices are adaptable and should be tailored to the specific context and requirements of each AI system.
- Implement Robust Data Governance and Security Policies: Implementing measures such as encryption, authentication, access control, and backup protocols is essential to protect data from unauthorized or malicious access, ensuring the integrity of AI systems.
- Maintain Data Quality and Integrity: Rigorous validation, verification, cleaning, labeling, and annotation processes ensure that the data used by AI systems is accurate, complete, consistent, and reliable, which is critical for their performance and trustworthiness.
- Utilize Data Minimization and Anonymization Techniques: Techniques like aggregation, masking, pseudonymization, and differential privacy help reduce the amount and sensitivity of data collected and processed, protecting the identity and privacy of data subjects.
- Develop Secure and Resilient AI Architectures and Algorithms: Employing methods such as encryption, obfuscation, and adversarial learning defends AI systems against various cyberattacks, maintaining their robustness and reliability.
- Incorporate Ethical and Human-Centric Design and Evaluation Principles: Frameworks that emphasize fairness, accountability, transparency, and explainability ensure that AI systems align with the values and rights of users and stakeholders, fostering trust and ethical use.
- Set Up Cross-Sector and Multi-Stakeholder Collaboration Platforms: Initiatives like standards, guidelines, codes of conduct, and best practices enable the sharing of knowledge, experience, and resources, fostering trust, consensus, and cooperation on AI security issues.
AI and data security are fundamentally interconnected, with AI systems heavily reliant on secure data usage. Addressing the security of both AI and data extends beyond technical solutions, encompassing social, ethical, and legal dimensions. This multifaceted challenge demands a collaborative effort from developers, providers, users, and regulators. By following best practices, we can use AI's transformative potential while mitigating associated risks, fostering a safe and ethical AI ecosystem that benefits all. A proactive and comprehensive approach to AI security ensures that we maximize AI's advantages while minimizing potential harms, paving the way for a future where AI enhances industries and improves lives responsibly.
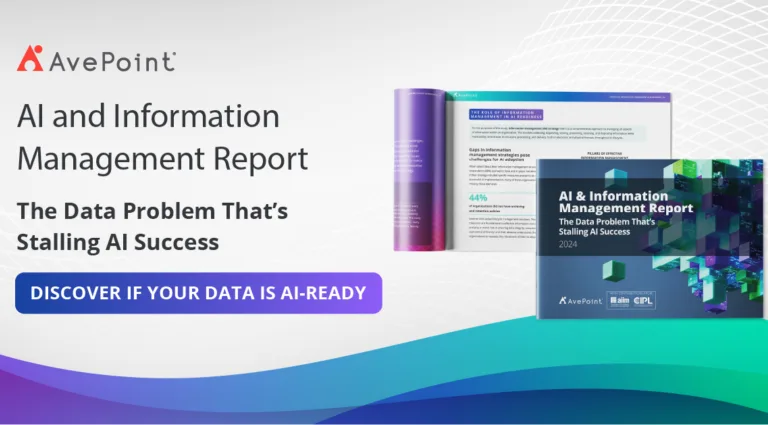