In the era of powerful AI models like ChatGPT, there's this notion that data may not be as vital as once thought. After all, these models can produce seemingly sophisticated outputs from a simple prompt. But dismissing the value of data would be dangerously misguided.
The truth is that data remains the backbone of AI and the fuel for innovation. Without robust, high-quality data, even the most advanced AI systems are little more than parlor tricks. From the training data that powers language models to the operational data that feeds decision intelligence, good data is fundamental.
But data's impact radiates far beyond just AI. In today's insight-driven climate, companies that harness the power of their data assets gain a strategic advantage. Used effectively, solid data enables new products and services, smarter operations, and evidence-based strategies that can reshape industries.
In this article, we’ll explore the vital role that data plays in driving AI and innovation, and the tremendous opportunities you can unlock by prioritizing quality, robust data.
Data is AI’s Secret Sauce
Let’s address the elephant in the room: does data really matter for AI success? Yes, it absolutely does.
The ability of AI models to learn patterns, gain knowledge, and generate intelligent outputs hinges entirely on the data they are trained on. Even the most sophisticated language models are essentially souped-up pattern matchers — studying vast datasets of digital text to statistically determine what word sequences make sense.
Remember, many public AI tools like ChatGPT, which seemingly pull valuable information from thin air, are actually crawling the internet for answers; their skill lies less in creating novel output and more in procuring relevant information and synthesizing it intelligently.
Think of it this way: in 2023, the world created around 120 zettabytes (ZB) of data, or roughly 337,080 petabytes (PB) per day. That's an immense amount of data for AI models to learn from!
Zoom out from natural language AI, and you'll find data is equally vital for other domains like computer vision, speech recognition, recommendation engines and more. These models rely on ingesting and learning from massive, labeled datasets of images, audio files, customer interactions and so on.
So, while AI systems can now achieve remarkable feats, data remains the indispensable raw material that allows them to acquire knowledge and build intelligence. The quality, quantity and curation of data are fundamental for developing AI capabilities that provide real-world value.
Clean Data is Make-or-Break for AI
The quality and relevance of data training AI is critical. Models trained on low-quality, biased or insufficient data will simply underperform, make mistakes, or propagate societal biases in their outputs. Some studies show over half of the outputs from powerful language models like ChatGPT already contain inaccuracies — a problem compounded when the models ingest flawed training data.
The consequences of deploying biased or error-prone AI can be serious – from alienating customers to perpetuating harmful stereotypes to making flawed, high-stakes decisions – and these risks will only escalate; Gartner predicts that 60% of government AI and data analytics investments will directly impact real-time operational decisions by 2024.
Whether it's optimizing supply chains, identifying health risks, or detecting cyber threats - data is what allows AI algorithms to build real-world knowledge and learn to make intelligent predictions. Without plentiful, properly curated training data, even the most advanced AI models are little more than proficient guessers unable to tackle real business problems.
This underscores the importance of robust data management practices. Despite the importance of quality data being clear, many companies don’t prioritize it before jumping headfirst into AI adoption. In fact, an AvePoint study found that 52% of organizations experienced data quality challenges when implementing AI.
Discovery How to Overcome the Data Readiness Gap in Your AI Strategy: Get Started Now
AI-Ready Data is Good Business, with or without AI
While data powers cutting-edge AI capabilities, its impact radiates far beyond just machine learning models. Organizations that can effectively harness their data resources gain a significant competitive edge in ways that they might not necessarily expect.
Gartner calls this concept “Data-Driven Innovation (DDI).” It involves “the use of data and analytics to develop or foster new products, processes, organizational methods and markets…[driving] both the discovery and execution of innovation, achieving new business models, products and services with a confirmed business value.” In other words, Gartner argues that, when organizations adopt a data-driven solution to a particular problem or need, they put themselves in a position to innovate in new and unexpected ways. This could include the development of a new solution, the emergence of a new use case for a particular solution, or the discovery of an entirely new pain point. Data can help you solve known challenges, but it also helps solve and discover new ones, too.
Like other data-driven technologies and practices, DDI can apply to generative AI. While an organization might originally adopt AI to strengthen customer support, for example, that same organization may find that AI creates opportunities to streamline other parts of the business. Data and information management is one area where companies may see positive trickle-down effects from AI. By cleaning up enterprise data to prepare for AI, organizations are making themselves leaner and more effective. A recent study from the Harvard Business Review, for example, finds that data-driven companies tend to be more profitable than companies that don’t practice data-first strategies.
What Next?
While some may be questioning data's relevance amidst the rise of powerful generative AI capabilities, the truth is that high-quality data has never been more vital — both for powering advanced machine learning models and fueling innovation more broadly across industries.
Companies that treat data as a strategic asset and masterfully harness their data resources will pull ahead of competitors. Conversely, those that neglect their data pipelines, practices and quality do so at their own peril — handicapping capabilities from AI breakthroughs to data-driven operational efficiencies.
As AI and automation mature, success will depend on an organization's ability to establish strong data foundations and governance to drive these technologies securely and reliably at scale. From the C-suite to data practitioners, companies need to prioritize data excellence as a core business capability. It underpins every strategic agenda from customer experience to operational resilience.
In the era of generative AI, big data and accelerating digital transformation, developing data mastery is vital for maintaining a competitive edge. Those that invest in getting data right will be well-positioned to capitalize on the disruptive possibilities that intelligent technologies present.
Dig deeper into the role of data and information management in AI success. Tune in now.
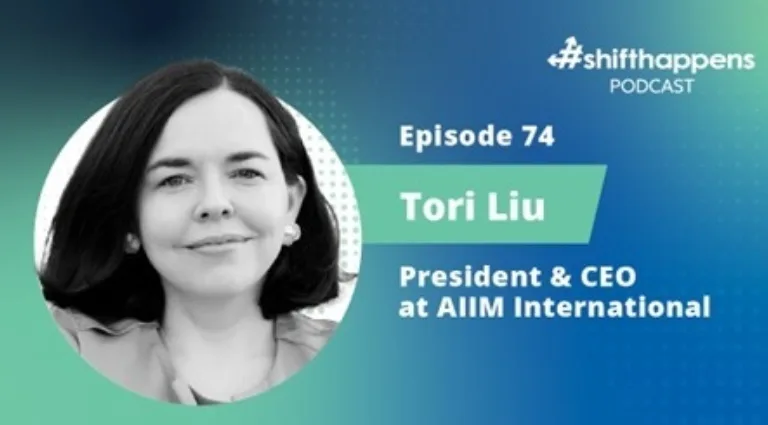