Episode 92: Moving Beyond AI Hype to Responsible Implementation
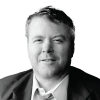
Organizations face new challenges in a more complex environment accelerated by digital transformation and generative AI. Succeeding in this environment requires more than just implementing the latest tools: It demands strategic thinking, ethical consideration, and a focus on real value creation.
In this #shifthappens episode, Dux Raymond Sy, Chief Brand Officer at AvePoint, and Jon Reed, Co-Founder of diginomica, discuss responsible AI implementation, the gap between vendor hype and practical reality, and the importance of education in successful AI deployments. Jon shares his perspective on enterprise adoption and cutting through the challenges to maximize opportunities.
Enterprise AI Reality Check
When many expected a return to “normalcy,” the technological landscape emerging from the post-COVID pandemic is anything but straightforward. Jon describes it as a “Darwinian” environment complicated by global conflicts, supply chain disruptions, and macroeconomic headwinds. Against this backdrop, the surge of generative AI promises transformative change, but realistic implementation requires measured and responsible strategies.
He notes that while the pandemic accelerated digital transformation, particularly around collaboration tools and cloud adoption, it also artificially inflated software spending. Despite the massive buzz around AI, he observes that enterprise spending has not followed the same market growth trajectory as vendor marketing or industry analysts might predict.
Jon argues that vendors are pushing technology aggressively when they don’t have to. Enterprises are already interested in adopting AI, and what’s really needed is education and an understanding of the pros and cons of AI deployments. “I think where vendors have fallen short quite a bit is by not talking enough about how they do that and how they protect the data differently than a consumer model. One of my big points is that you have to get into education. A lot of customers really want a walk-through to understand the data flow,” he explains.
The Critical Role of Data Quality and Security
One of the most crucial insights from this episode centers on the need for high-quality, relevant, and up-to-date data for AI to have the positive impact businesses expect. As organizations rush to implement AI solutions, many overlook the fundamentals of their data estate and security concerns around data access and sharing.
Jon emphasizes that enterprises must prioritize two fundamental considerations:
- Data Infrastructure: Organizations need to assess their current data landscape to ensure it is clean and well organized as well as have a clear understanding of how AI can integrate structured and unstructured information.
- Data Security and Responsible AI: As regulatory environments evolve, companies must implement robust data protection architectures, understand AI’s data flow and potential risks, and stay informed about emerging regulations.
Jon touches on the evolving industry-specific regulations for AI, particularly the EU AI Act and its implications, copyright concerns for creative content, and data privacy requirements. He highlights that enterprise implementations can actually be more secure than consumer applications, noting “The good news is that I do think a lot of vendors, including Microsoft and many others, are working hard on responsible AI architectures,” he adds.
Purpose-Built AI: The Real Opportunity
The most promising AI implementations are highly specific, purpose-built programs rather than enterprise-wide, one-size-fits-all solutions. Jon shared several compelling use cases of successful AI implementations, “For example, you can have much smaller models that are custom to a particular customer. [The customer] technically still owns all of [their data] and the data never really leaves their premises. It never goes into the large language model. If you use a large language model, the customer data is only manipulated through a prompt and some type of technique like retrieve log-menu generation. So it never becomes part of the model set at all.”
A particular case is Loop Insurance’s customer service transformation. The results of their carefully implemented AI support bot include:
- 3x increase in customer self-service rates to more than 50% automated resolution
- 75% positive customer satisfaction rating for their AI assistant
- 55% decrease in email tickets
What made this a success story is the focused approach to AI-controlled architecture, which was limited to support issues only. Loop Insurance implemented well-trained escalation protocols and high-quality support documentation. Most importantly, there was no headcount reduction, only role enhancement.
The Human Element in AI Implementation
A significant theme throughout the conversation was maintaining and enhancing the human element in AI implementations. “Companies must make it clear what their strategy is to their employees. I think companies that are more thoughtful and vocal about how [AI] is going to help serve customers better and help your career will have a lot more success than the ones where it's like, wait, what's going to happen? Am I going to be automated out of existence? Cause it's a natural question to ask and not everyone understands how this technology is evolving.”
Responsible AI implementation focuses on workforce enhancement. Jon stresses the opportunity to redeploy and enhance human capabilities by creating opportunities for upskilling and retraining employees for higher-value tasks. For example, moving support agents into sales roles for upselling and customer interactions.
Contrary to apocalyptic narratives, Jon believes the most successful AI strategies will view technology as a collaborative tool, not a headcount replacement.
Practical Steps for Responsible AI Adoption
Jon provides a valuable framework for organizations considering enterprise AI implementation:
- Assess AI Readiness: Evaluate data infrastructure, assess data quality, and consider both structured and unstructured data needs.
- Choose the Right Partners: Find trusted providers that also embrace safe and responsible AI implementation.
- Start with Practical Use Cases: Focus on clear return on investment opportunities, beginning with well-defined, limited scope projects and building organizational momentum through early successes.
- Develop a Clear Narrative: Communicate how you will implement AI that resonates with your employees, customers, and executive leadership.
- Foster Experimentation: Create safe environments to test new AI applications, encourage use-case ideation, and balance practical implementation with innovation.
While technology holds tremendous promise, success depends on realistic expectations, a focus on data quality, a commitment to responsible implementation, clear communication with stakeholders, and a balance between practical results and innovation.
The future of AI in enterprise is not about replacing humans or chasing the latest hype, but about thoughtful implementation that enhances human capabilities and delivers real business value.
Relevant Episodes
- Episode 93
AI in Accounting: Addressing Resistance to Adoption and Change Management
- Episode 91
- Episode 90
- Episode 89
Women in Tech and the Role of AI in Diversity, Equity, and Inclusion
- Episode 88
- Episode 87