Building AI Excellence: A Framework for Data Quality and Classification
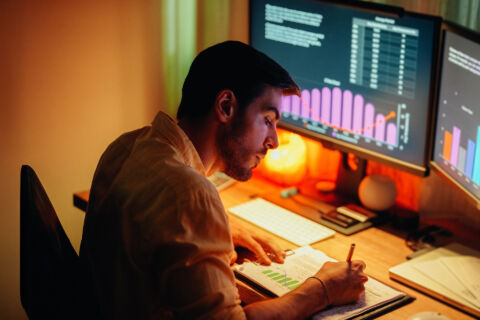
We are all realising that data is the lifeline of organisations — a fact underscored by an increasing dependence on AI systems harnessing this data in an effort to improve operational efficiencies whilst producing quality output.
The use of generative AI (GenAI) has increased from 55% in 2023 to 75% in 2024. With an estimated global spend on AI by 2028 set to reach US$632 billion, this growth only amplifies the need to take full advantage of this technology — for the right reasons. This acceleration aligns with the findings from AvePoint's 2024 AI and Information Management Report, detailing how organisations want to achieve specific business outcomes, such as improved efficiency and productivity (61%), increased data insights and accuracy (54%), and enhanced decision-making (51%).
While this change in business priorities indicates a clear commitment to AI-driven transformation, it also highlights critical and underlying foundations needed to realise the value of AI, namely the need for a robust data quality and classification framework. But what does this actually mean?
Let me paint a picture: Have you ever tried to find a wooden spoon in that dreaded second drawer in your kitchen, only to find one that’s broken or not even a wooden spoon; this is frustrating and not very helpful. If we take the same approach to our data, AI outputs are only as good as the data within the systems. This raises questions about the relationship between high-calibre data and AI, and how businesses can ensure their data assets are properly structured, accurately labeled, and consistently maintained to deliver on AI’s promised benefits.
In this blog, we will talk about some important considerations on how ensuring high-data quality and accurate classification can help effectively accelerate AI initiatives.
Best Practices for Ensuring Data Quality
How do we ensure data quality? The first step is to understand what you have, and then how much of it is redundant, obsolete, or trivial (ROT). Without a strategic approach, systems often hold irrelevant, inactive, and unstructured data, leading to a series of unfortunate events, whereby GenAI pulls old information or creates hallucinations, reducing the quality of GenAI outputs and increasing risk to the business.
So, what do we do? Organisations must ensure to follow these practices:
- Data accuracy and consistency. Implementing data validation, cleansing, and standardisation leads towards a high-quality data estate. By doing this, our data becomes standardised, accurate, and complete, meeting the defined standards before it is fed into AI systems.
- Data integration and interoperability. The smooth integration and interoperability of data across multiple systems goes hand-in-hand with boosting data quality. However, we need to identify the source of truth; if we have multiple systems with data points, how do we ensure accuracy if we don’t understand the datasets? When we truly engage in interoperability, systems will access and exchange data without friction (think Dataverse) and improve AI performance and insights.
- Auditing and monitoring. We may at times think our data is “clean,” but while this may have been the case at a point in time, if we are not proactively reviewing through regular data audits, implementing continuous monitoring, and remediating data deficiencies, this can lead to poor data quality — impacting GenAI outputs.
- Enacting data disposal. While the foundation of your data and a proactive approach is essential, understanding how long you need to keep data is just as important. Data that can be defensibly disposed of in accordance with retention and disposal schedules and/or applicable legislation (noting that there are a lot of things to contend with when it comes to legislation, but that’s for another time) will reduce the quality of GenAI’s performance using legacy information to generate inaccurate responses.
Effective Data Classification Strategies
What does classification have to do with AI? Not only does classification assist in the defensible deletion of information, but it also ensures proper governance is applied based on what the information is about and the sensitivity of the information, thereby limiting GenAI from accessing the wrong information.
With this approach, the following are the key things to consider:
- Aligning classification with business objectives. There are industry standard classification schemes such as Keywords for Council or Keywords AAA, a thesaurus of common terminologies that uses the keyword classification method and was developed by the New South Wales State Archive and Records. As a baseline, they provide validation in “common things,” but the likelihood they align with the strategic and/or operational goals and philosophies of your organisation is unlikely. The approach in which the classification is developed needs to align with your organisation. And while you can gather information from other organisations of a similar type, we’re not talking a one-size-fits-all here to get the optimal outcome. Let’s take the analogy of the kitchen drawers (I do love a good analogy); the requirements for the top three drawers in my kitchen are to get easy access to utensils when cooking, but if I were to just take what my mother has in her top three drawers, I could be putting coffee in my top drawer and cookbooks in my third drawer — while the former approach works for her, it doesn’t work for me. So, think about your business and what the right approach is for you based on your needs.
- Understanding data sensitivity and value. The value and importance of security cannot be underestimated; based on the notifiable breaches from the Office of Australian Information Commissioner between 2020 and 2024, 32% of data breaches were a result of human error. Effective categorisation is imperative and is based on organisational requirements through undertaking an assessment of the data based on its confidentiality, integrity, and availability. This framework analyses the risk of the information, and a structured classification system ensures you manage your data according to its significance and risk profile.
Impacts of Poor Data Quality and Classification
As we’ve described throughout, poor data quality and ineffective classification can lead to significant short- and long-term consequences, but the lack of trust in your organisation’s data can erode employee confidence in AI, therefore impacting AI initiatives. IBM notes that 42% of their surveyed respondents reported feeling that their organisations did not have enough proprietary data to be effective with GenAI.
Unreliable outputs from AI systems can compromise operational achievements such as leveraging correct market insights and customer behaviours, which leads to misguided campaigns, costs, and resources being spent on fruitless adventures.
In the realm of data security, the stakes are even higher, as inadequate data classification can expose information, leading to insider threats and data breaches. Failure to comply with information protection regulations due to poor classifications can also result in significant fines and legal repercussions. Moreover, there is a reputational risk for organisations, with customers losing trust where it’s proven that these organisations cannot protect their sensitive data.
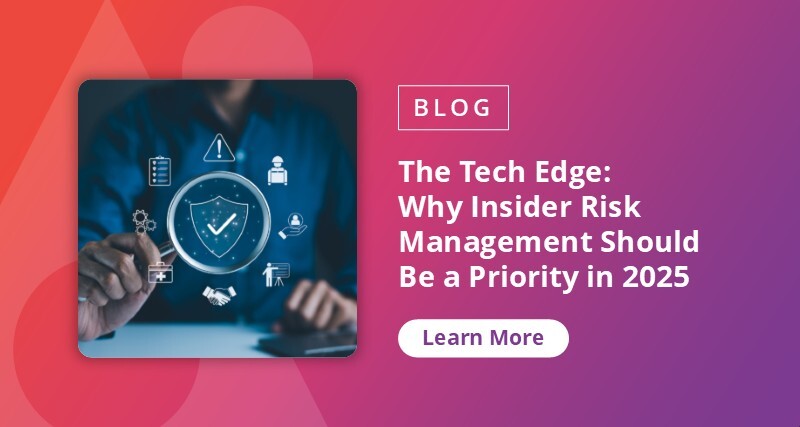
Organisations must turn to robust data governance practices, continuous improvement initiatives, and clear data policies. They must also enforce regular audits and conduct regular employee training on the importance of data hygiene. Indeed, continuous monitoring and updating of data management practices empower organisations to ensure ongoing compliance and security.
From Quality Data to Transformative AI
High-quality, properly classified data is, as we know, the cornerstone of successful AI adoption. Implementing a solid data governance and classification framework will not only enhance AI performance but also secure, scale, and transform a digital workplace.
As organisations continue investing in AI technologies, those with robust data governance frameworks will realise substantial competitive advantages, while those neglecting data quality will face escalating risks and diminishing returns.
Looking ahead, AI will continue evolving at breathtaking speed, and organisations that cultivate a culture of continuous data improvement today will be best positioned to adapt to tomorrow's innovations. The future belongs to those who recognise that exceptional AI outcomes begin with exceptional data management — making the investment in data quality not merely a technical requirement, but a strategic imperative for sustained success.
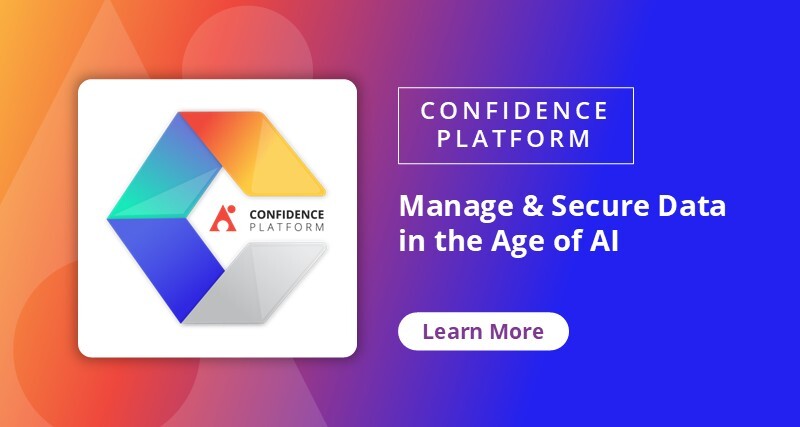
Janine Morris is an experienced information management professional who helps organizations reduce information chaos and improve employee experience while meeting regulatory and compliance requirements. She holds a Master's degree in Information Management and her professional approach and passion have earned her solid recognition in the industry, including being recognized as a Membership Fellow (FRIM) and serving as a former board director and branch president of RIMPA Global.