The Critical Link: The Role of Data Governance in Digital Maturity

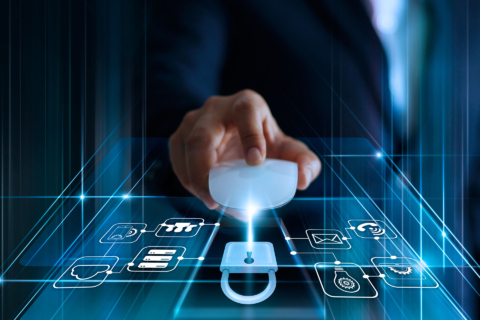
In an era where digital prowess defines success, digital maturity determines which organizations thrive and which fade into obsolescence. Both Gartner and Microsoft offer digital maturity models that organizations can use to score themselves. Gartner’s model categorizes organizations into the following five levels:
- Organizations in the first and second levels lack fundamental strategies, face high risk, and see low AI returns.
- Those in the third have enhanced security and efficiency but are not yet optimal.
- Finally, those in the fourth and fifth tiers boost their data, co-create solutions, empower their workforce, and are able to reach maximum security and productivity.
Without a credible set of criteria like those offered by Gartner and Microsoft to benchmark the success of their digital transformation efforts, organizations risk fragmented, failed initiatives and might not fully meet their growth targets, ultimately missing out on the value of their technological investments like AI tools.
Challenges to Digital Maturity in the Age of AI
Today, digital maturity demands the successful deployment of AI tools across a workforce, with Harvard Business Review reporting that 89% of large companies globally have both digital and AI transformation underway. However, the same report found that these companies have only captured 31% of the expected revenue lift and 25% of the anticipated cost savings. Although AI’s ability to vastly improve business outcomes is widely recognized, it’s clear that organizations face the more complex challenge of crafting and implementing a sustainable AI strategy that delivers real value.
More importantly, this challenge extends to a lack of sufficient security, technological support, and internal procedures to execute AI safely. In our 2024 AI Information and Management Report, we found that 45% of organizations experience unwanted data exposure during initial AI implementation. It’s no wonder, then, that alongside data quality and categorization, data privacy and security are top concerns among companies.
The fact that many organizations around the world still face data-related challenges when implementing AI underscores the likelihood that many businesses are skipping crucial steps to achieve maturity. One possible reason for missing these steps is the belief that simply introducing new technology, such as AI, will automatically lead an organization to digital workplace maturity. This, however, could not be farther from the truth.
Before organizations can achieve digital workplace maturity, they must first ensure their workforce is properly trained and ready to take on AI. People-focused enablement training helps ensure safe and efficient AI use.
A workforce that leverages AI also needs quality data — meaning data that is relevant, updated, and classified. By now, it is no secret that data is the lifeline of large language models (LLMs) that power AI systems. With redundant, obsolete, or trivial (ROT) data feeding AI systems rather than quality data, an organization cannot expect relevant and meaningful results.
To effectively train your employees on how to best leverage AI, therefore, you must first secure and optimize data on a technical level. That means bolstering data governance and going beyond conventional notions of data security to advance your digital maturity.
Limitations of Traditional Data Security
In the rapidly evolving landscape of AI systems and their integration to modern workflows, traditional notions of data security will not suffice. The traditional scope of cybersecurity, for example, prioritizes the protection of data from unauthorized access and external threats, such as malware or ransomware groups, phishing, distributed denial-of-service (DDoS) attacks, and zero-day exploits where cybercriminals exploit software vulnerabilities that have not yet been patched.
Cyberattacks in the age of AI, however, not only expand the attack surface from the perspective of external cyberthreats but also emphasize possible data exposure that may occur from inside an organization. For example, while it is true that the large datasets required by AI systems to operate are attractive to cybercriminals, encouraging a new slew of social-engineering methods, employees might also accidentally access sensitive information that is not relevant to their job function if their organization lacks the necessary security guardrails around that information.
Microsoft 365 Copilot’s integration with a wide range of data sources may, without user knowledge, inadvertently lead to AI-generated data that includes sensitive information like confidential business data or an employee’s private information. For example, an HR employee might use an AI tool to draft a report, without realizing that the tool might use sensitive details from the organization’s HR database, which would include salary information, performance reviews, or personally identifiable information (PII).
When it comes to AI-generated information, therefore, data security alone cannot protect data while also ensuring its quality. In contrast, data governance is the all-inclusive framework that defines and safeguards confidential, personal, and sensitive data types while also ensuring data quality, security, and availability.
The Role of Data Governance in Digital Maturity and AI
Traditional notions of data security, therefore, are not enough for an organization to achieve digital maturity. Instead, organizations need data governance – the comprehensive process of managing and protecting organizational data assets by establishing policies and standards – to ensure that they achieve their digital maturity goals.
AvePoint’s Confidence Platform follows a multilayered approach to data governance to effectively scale AI, eliminate data silos, and deliver holistic resilience with these vital steps:
- Identify and protect. When a user creates a document, the Confidence Platform identifies and classifies the data, ensuring content is properly protected during migration.
- Optimize. AvePoint uses AI solutions to monitor changes in risk, permissions, and data value. The Confidence Platform automatically protects new users and data, applying information lifecycle policies efficiently.
- Defensibly destroy. AvePoint enforces governance for critical processes. Automated access reviews and data lifecycle policies ensure consistent compliance. Storage is optimized by managing necessary data, reducing IT resource burden.
- Drive insights. The platform helps organizations analyze business value and data consumption patterns. Insights are mined to support strategic decisions. High-traffic data loads receive enhanced protection to prevent breaches.
- Responsibly collaborate. Secure file sync and share options are provided for external teams, ensuring that sensitive data remains protected. Files can be stored in secure data rooms to prevent unauthorized access. Granular item-level security measures are implemented to eliminate risky sharing links and enhance overall data security.
These comprehensive security and governance strategies behind AvePoint’s Confidence Platform lay the foundation for the analytics-driven insights that are crucial for organizations to achieve their digital maturity goals.
The Importance of Analytics in Digital Maturity
For organizations eager to attain digital maturity, analytics on sensitivity labels, frequently visited sites, and permissions are critical to executing data governance.
To gain insights around data governance and engagement, organizations can leverage the Confidence Platform’s analytics capabilities:
- Identification of sensitive data. In addition to identifying sensitive files that may be overshared, track who has access to it and pinpoint if any external users pose a threat — in short, see what people are doing with what in an organization.
- Locating high traffic areas. Recognize high-traffic areas such as SharePoint sites, Teams Channels, and Viva Engage Communities within an organization. As these areas likely provide top-notch resources and value to users, they require more robust protection.
- Data obfuscation. Configurable protection of PII ensures that sensitive data remains anonymized while also providing context to organizational engagement behaviors.
- Enforcement of security rules. Administrators can define the access level of sensitive data and ascertain that business users have access only to the reports that are business-crucial for them.
- External benchmarking. Executive leadership can optimize the value of their AI adoption when they gauge their AI strategy against those of other global companies. External benchmarking capabilities empower IT and executive leaders to gauge how their adoption stacks up to set realistic targets.
Learn more about AvePoint’s Confidence Platform and leverage analytics to boost your organization’s digital maturity today.

Andie is a Content Marketing Specialist at AvePoint, covering migration, employee experience, and education technology. With 15 years of experience in B2B communications and content editing, Andie creates content that helps businesses realize the value of their technological investments.