Overcoming the Data Readiness Gap for Successful AI Implementation
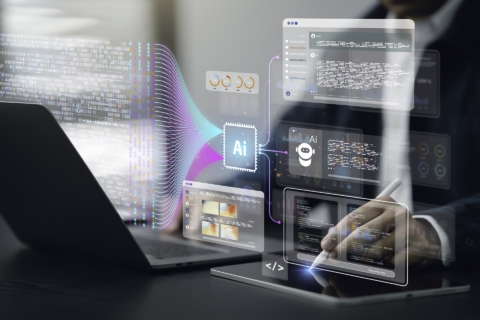
Data is a critical aspect of an organization’s digital transformation journey, in particular AI. Much like a car cannot run without fuel, AI applications require continuous data input to become and stay functional. As volumes of data created, captured, and replicated keep growing fast, it has become mission-critical for organizations to get an accurate handle on their information management strategies for a successful AI implementation.
Results from the AI & Information Management Report 2024 highlight a significant gap between perception and reality regarding data readiness for AI adoption. About 80% of organizations believe their data is ready for AI; however, 52% faced challenges with data quality and categorization during the implementation process.
This data readiness gap is becoming even more crucial to address as generative AI ushers in a new wave of AI adoption. IDC predicts that generative AI will trigger a market transition to “AI everywhere,” which will define the next frontier of the digital business journey. With powerful AI capabilities becoming widely available, new opportunities and significant risks arise; to navigate this transition successfully, every organization will need to chart its path to optimize and secure data responsibly.
How do you overcome the data readiness gap for successful AI implementation?
Challenges Causing the Data Readiness Gap
While organizations recognize the importance of data readiness for successful AI implementation, many still face significant hurdles in this area. Some key challenges that organizations need to overcome are:
- Volume. Most organizations’ information management strategies cannot keep up with the increasing volume of structured and unstructured data, with 64% managing at least 1 petabyte (PB) of data and 41% managing at least 500 PB. The more data an organization has, the greater burden it faces — in terms of costs, risks, but mostly importantly, management complexity, making it difficult to ensure high-quality data for AI training and us.
- Accessibility. Organizations often scattered across various repositories within an organization. In fact, 87% of organizations report to storing data in the cloud, but a significant portion also have data in self-hosted storage (51%) and physical documents (46%). Creating effective training models with AI algorithms involves access to all relevant information, and it can be challenging for AI to aggregate and analyze when data is stored in disparate formats and fragmented locations. If data is stored on-premises or in physical formats, AI may not have direct access, hindering its ability to utilize the information effectively.
- Quality. AvePoint’s research also found that 50% of organizational data is over five years old, likely containing redundant, obsolete, and trivial (ROT) data. Inaccurate or outdated content compromises AI-driven insights that can adversely impact decision making and business outcomes. High quality data means complete, consistent, and timely information.
Given the complex data landscapes organizations operate in, it is essential to establish regular checks and balances across the information lifecycle. This continuous process ensures data availability, relevancy, and accuracy for AI to extract value fit for your purpose and enables AI to recognize patterns and refine its output to your evolving needs. Failing to implement basic measures and confirm your data is ready for AI increases the risk of misleading and erroneous decisions affecting your customers, reputation, and overall operations.
Read more about the challenges stalling the success of AI in our free eBook:
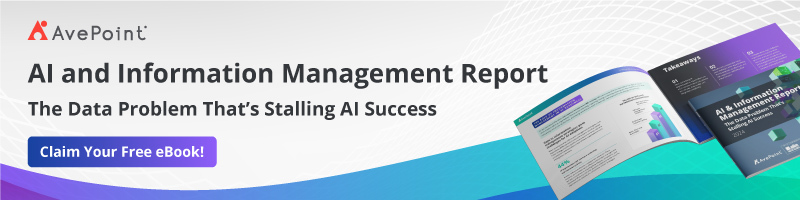
3 Strategies to Navigate the Data Readiness Gap
To navigate the data readiness gap for AI success, organizations must deploy targeted strategies aimed at optimizing their data infrastructure. Here are three essential strategies to consider:
1. Assess your data to make good decisions
Understanding your organization’s data landscape is the first step towards making informed decisions that can improve the quality of your data.
Begin by assessing your data, determining the data types, storage locations, and data structuring in your data ecosystem. This deep dive is critical to gaining the knowledge that will help businesses lay the foundation for an effective data cleanup and preparation necessary for accurate AI input and output.
AvePoint Opus, an intelligent information management solution with AI-powered capabilities, provides organizations with unprecedented visibility into their data landscape. This comprehensive view of your data assets allows you to understand what data exists, where it resides, how it is structured, and what its potential business value is, making it easier to determine ROT from necessary data when you begin to clean up your repositories.
2. Clean up your current repositories
AI models thrive on high quality and well-structured data, which means organizations must ensure that their data is clean, secure, and accessible in a format that AI systems can utilize effectively.
The key is to consolidate all relevant data into a centralized location for AI systems to enhance efficiency and reliability of results. Having a unified view simplifies AI’s search instead of navigating complex databases. It also reduces errors by allowing quick access to a single source for tracing data issues, rather than relying on disparate systems.
Discover how you can unify and enrich your data to enhance the AI experience.
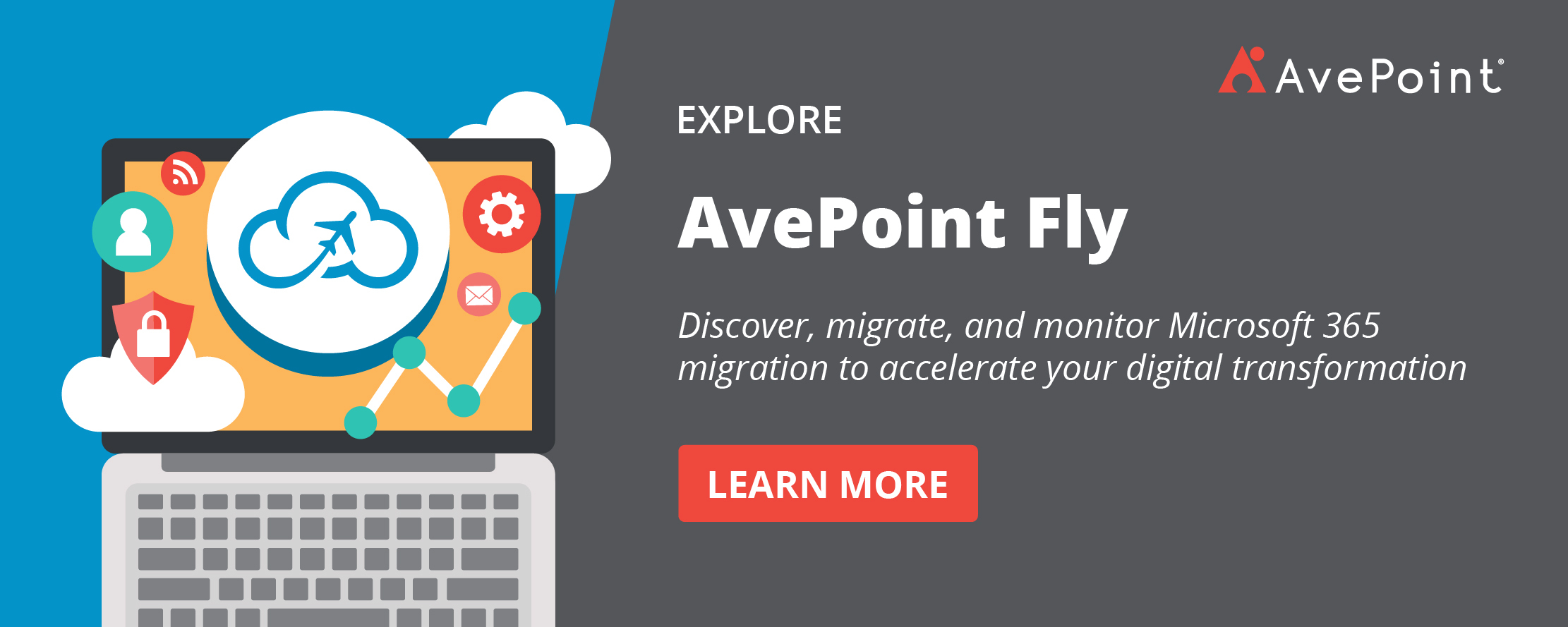
Data cleaning involves eliminating ROT data and inconsistencies, while data preparation involves transforming raw data into a format suitable for analysis ensuring that the remaining data is properly classified and categorized.
You can use AvePoint Opus to take direct action based on the results of your landscape audit, archiving, and deleting ROT content. This not only helps to clean up your data sets for AI consumption but also frees up valuable storage space, reducing your overall storage costs.
3. Automate data management processes
Data cleaning and preparation is not a one-off activity and should be part of an ongoing maintenance process. Rather than depending solely on manual and outdated rules, automating processes can empower organizations to streamline and optimize various aspects of the information lifecycle in preparation for AI.
AI can drive information management solutions to initiate tasks such as data classification, preservation, or purging based on evolving policies and business requirements. This enables organizations to keep up with the constantly expanding volume and complexity of data.
AvePoint Opus can automate information management best practices to streamline processes and AI readiness. By auto-classifying content as it is created and simplifying the application of retention and disposal rules, AvePoint Opus helps you keep a handle on your data repositories, taking control and ensuring quality, no matter how much data grows.
Explore how organizations can take control of their data and transform disconnected repositories into an intelligent information ecosystem.
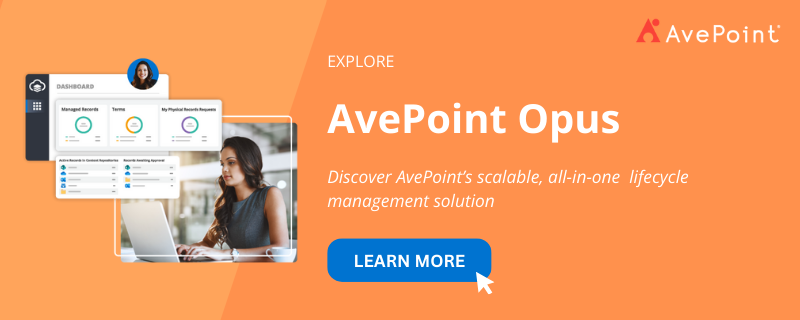
These measures work best when part of a larger information management strategy. If they are not part of your organization, you are not alone. According to the AvePoint study, 77% of organizations acknowledge they must implement new information management measures tailored to the AI evolution.
The research shows those willing to adapt have the highest levels of confidence in their AI-ready data. These measures not only provide valuable insights needed to improve efficiency and data quality, but also reduce time and resources by removing unnecessary processes in data-driven decision-making.
Data Readiness in AI Implementation
As AI continues to evolve rapidly, organizations must also be prepared to adapt their information management strategies to keep pace with new developments. They should increasingly explore the processes, skills, and technologies required to leverage information and data to achieve better business outcomes. It is not only about implementing the “latest and greatest” technology but more about building a solid foundation to guarantee that data is secure, organized, and easily accessible.
By taking a proactive approach to assess, clean up, and automate data management processes, organizations can maximize the benefits of AI that lead to cost savings through optimized storage and improved compliance. Embracing an information management strategy is crucial for organizations to capitalize on AI’s transformative potential.
Unlock the power of AI for your organization's information management needs. Watch AvePoint’s webinar as we dive into these emerging and game-changing technologies today!
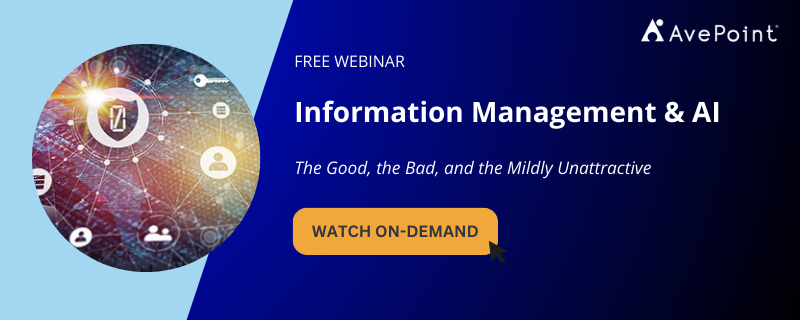
Amabel Palencia is a content specialist at AvePoint, covering information lifecycle management and artificial intelligence (AI). A journalism graduate, she has 15+ years of experience in project management, customer engagement, communications, and content marketing strategy for B2B campaigns.