The Tech Edge: The Future of Ethical AI Is Possible

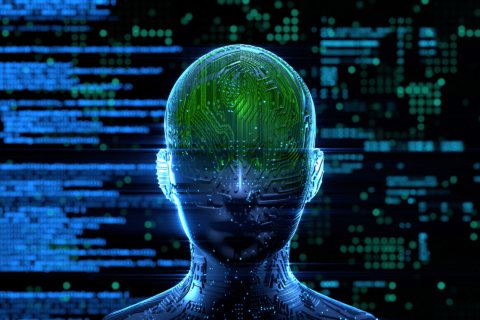
One of the first people I was fortunate enough to interview for in The Tech Edge was Tori Miller Liu, President and CEO of the Association for Intelligent Information Management (AIIM). Tori shared many incredible things, but here's something that made me really stop and think during our conversation. Tori pointed out that AI doesn’t really make errors or “hallucinate” in the way we think. It is doing exactly what it’s programmed to do — giving us the most statistically likely answer based on its training.
Just as a seemingly promising casserole recipe can yield unexpected outcomes (and my goodness, have I had some unexpected results — note for the future, don’t tip an entire bottle of wine and hope everything works!), AI can provide responses that appear ideal but actually may not be accurate in real-world applications because it’s looking for things that are statistically more likely, which doesn’t always equate to accuracy. So, let’s explore in more detail the advancements in AI technology, its applications, and ethical considerations we need to make when we’re choosing to implement it in our organizations.
Why Your AI Isn’t Actually Making ‘Mistakes’
AI is no longer a buzzword but a practical solution for solving real business problems. However, 95% of organizations faced challenges during AI implementation, primarily due to issues related to the quality and organization of internal data.
Just as a cluttered kitchen complicates cooking, disorganized data can reduce the reliability of AI. Tori emphasizes the importance of data hygiene in AI implementation, noting, “You start with the fundamentals of your data because that is the key to AI readiness and success.”
When businesses feed important documents like customer contracts or invoices into AI systems, the stakes are much higher. Imagine sending out invoices where AI has accidentally transformed the numbers because the source of information is disorganized, unverified, or outdated.
AI functions similarly to a student who has memorized all the information from textbooks but may not fully comprehend the content. Therefore, it is important to consider carefully how AI is implemented in business to avoid the potential consequences of inadequate data management. AI might have all the data, but it can lack context and relevance for all that data leading to inaccurate outputs.
Cultivating Human Potential in the Age of AI
The inherent nature of AI errors is attributable to issues with data, be that inherent bias, out-of-date content, or disparate systems, which can result in poor outputs from these systems. The primary concern is the accuracy of AI outputs rather than their statistical validity. While something might be a statistically valid response, it doesn’t necessarily mean it’s accurate. Tori critiques the notion of AI “hallucinations,” asserting that AI algorithms operate according to their programming.
Therefore, it is essential for humans to evaluate and verify AI-generated information carefully and to be selective about the data input into AI systems. Consider what information you are willing to share and what should remain private. Determine who has authorization to use AI tools with company data and where the resulting outputs are stored.
Organizations should educate employees about AI capabilities and risks and the need for diverse and representative data sets to mitigate these biases. Critical analysis of AI-produced content should be a key concern for all organizations. They should also develop ethical frameworks and guidelines to govern AI use to ensure that AI systems align with human values and societal norms. For example, you don’t want to misuse copyrighted material through AI unknowingly.
The EU Parliament and the US government are developing guidelines, such as the AI Bill of Rights, while existing frameworks like DAMA International’s Data Management Body of Knowledge can be adapted for AI governance. The key principles of these guidelines include ensuring data transparency, interoperability, continuity, and accuracy.
Safeguarding Data Integrity and Transparency
In our discussion, Tori defined data integrity within information management as follows: “Data integrity really talks about the lifecycle that data and information have traversed... you’re really interested in the accuracy, completeness, and consistency of that data over its entire lifecycle from the point of creation to the point of transformation within the AI algorithm to the point of output.”
Data integrity involves tracking the lifecycle from creation to transformation within AI algorithms. Enterprises must understand how AI may alter data, especially when dealing with sensitive information like invoices or customer contracts. Ensuring algorithms are explainable allows users to comprehend decision-making processes, fostering trust and reliability.
Proactively identifying and preventing the uncontrolled use of sensitive content is essential. Security, privacy, and transparency are key components of ethical AI frameworks. As technologies evolve, maintaining ongoing dialogue and collaboration with stakeholders is crucial to addressing ethical challenges and protecting intellectual property and copyright.
The Importance of Ethical Considerations
By focusing on education and transparency, businesses can harness the power of AI while mitigating risks and maintaining trust with their stakeholders. There is no silver bullet but proceeding cautiously while not halting AI development is recommended. As Tori reminded us, “We need to be responsible and careful as we approach it.”
Ethical frameworks should also cover AI’s privacy implications, particularly how data is collected, stored, and used. This includes informed consent in decision-making based on AI-generated data. Acting in good faith is crucial, given the current AI technology’s “black box” nature.
3 Practical Steps You Can Take Today
After diving deep into Tori’s insights, here are three actions that can make a real difference in your organization:
- Invest in information management programs. To ensure data hygiene and understand the repercussions of feeding data into AI engines. Tori suggests creating a map of data entry points, transformation stages, storage locations, usage points, and archival/deletion protocols.
- Implement proactive protection standards. Tori stressed the importance of “proactively identifying, mitigating, and preventing the uncontrolled use of sensitive content.” This means clear data classification systems, access control protocols, regular compliance checks, and training programs for all users.
- Start small but start now. Don't get stuck in what Tori calls “analysis paralysis.” A starting point for leaders is educating themselves and their team about AI solutions and ethical frameworks. Move quickly to experimentation to avoid analysis paralysis: pick one small, low-risk process to experiment with, document everything you learn, and build your confidence before moving to more critical applications.
Looking Ahead
Start with these three steps, keep learning, and remember: the goal is not to be perfect, but to be purposeful and responsible in using these powerful new tools. Ethical AI requires robust data management practices and continuous oversight to ensure fairness and accountability.
The future of AI depends on humans’ ability to develop and implement ethical guidelines that ensure fairness, transparency, and accountability. By addressing these ethical concerns, we can harness AI’s full potential while minimizing its risks.
You can check out this episode and more here: The Tech Edge - Ticker.
Want to learn more? Read our blog entry, Overcoming the Data Readiness Gap for Successful AI Implementation.

Alyssa Blackburn is the Director of Records & Information Strategy at AvePoint, where she helps organisations achieve business value from their information. In her role, Alyssa provides records and information consulting services as well as system implementations, allowing customers to optimise the structure of their information to maximize business benefits while meeting data governance and compliance objectives. With 20 years of experience in the information management industry, Alyssa has worked with both public and private sector organisations to deliver guidance for information management success in the digital age. She is responsible for the development of AvePoint’s information management solution, and has been involved with implementing our records management solution with government agencies and commercial clients. Alyssa is actively involved in the information management industry and has spoken at a number of events including at Inforum 2016 in Perth. She has been published in the RIMPA IQ magazine and recently won the 2016 award article of the year for the RIMPA IQ magazine for her article titled, "Why you need to think differently about information management."